- Home
- Search
- Jonathan C Kao
- All Reviews
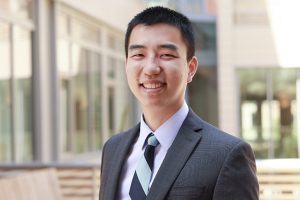
Jonathan Kao
AD
Based on 99 Users
Like everyone said, Kao is probably one of the nicest professors I've ever met. While some professors care only about research, it's clear that Kao genuinely cares about teaching. He wants us to learn in an intellectually honest way and grasp the material conceptually. He encourages everyone to come to office hours, he encourages people to ask questions, and he is so super approachable. He has a forgiving late day policy and will give class wide extensions if the class expresses a need. He wants everyone in the class to succeed. The material is challenging but he makes it super accessible and it's super interesting.
The class is 40% homework which sounds nice but I found the homeworks to be harder than the tests because they were long and they had us work on content we literally just learned. You MUST start early. I would say to start immediately, as homeworks are usually assigned Thursdays, do as much as you can over the weekend, and then go to the TA's or his office hours during the week to answer questions. It is very possible that you won't be able to do a lot of the homework alone/when you first look at it, but he and the TAs are VERY helpful and will guide you to the right answer. I like to think I'm not an idiot, and I absolutely could not have done these homeworks without going to office hours.
The rest of the class is 25% midterm and 35% final. After the midterm, he said that if we performed better on the final, he would replace our midterm score with our final score (he's super supportive and on the student's side!). As more Fall 2023 reviews come in, I expect people to say the midterm was easy and the final was hard. I don't know that I will completely agree. The midterm was extremely straightforward, as the TAs held a review session before the midterm that had problems that were almost identical to the real midterm. Thus, many people left after 40 minutes, and the median on the midterm was a 92. I think Kao reacted appropriately and adjusted the difficulty of the final to make it more challenging. I also think a lot of people were expecting the final to be just as easy and it was not. The content on the final is just in general more challenging, but we also weren't spoon fed the entire final like we were the midterm. Thus, many people freaked out after the final and said it was super hard, and I don't think this is super accurate. The final did have a much lower average than the midterm (in the 70s I believe) but I just feel like a 92 median is high in general. There was not a single question on the final that was completely out of left field -- I genuinely believe every question was fair game. Thus, main point is: go to office hours, go to TA review sessions, do EVERY SINGLE practice midterm/final/discussion/homework. And make sure you KNOW the material from a CONCEPTUAL STANDPOINT. To study for the final, I went through every single discussion worksheet, homework, and the past 4 years of finals (he provides them god BLESS) and made sure I understood everything. Also, on your cheat sheet, do NOT just put formulas. The tests test HOW YOU APPLY THE CONCEPTS. He will NOT just be like "State the convolution theorem :D (10pts)." So look at the midterm and final answer keys copy solutions of different types of problems, it will really help you out. Good luck, and really take advantage of what an amazing teacher he is!
The other reviews pretty much sum him up well. This class is really, really hard, but I don’t think anyone else in the department can teach it better than him. So, I’d highly reccommend
I love Jonathan Kao. His lectures are very clear—with amazing annotated notes. Concepts that may seem confusing—Kao has a unique ability to make them seem approachable and common sense-like.
In office hours, he is always willing to take questions, talk about the course, or just life in general. I've had great conversations with him regarding the existence of free will. Not many professors are that engaged with their students.
One minor criticism I have of Kao is how he takes questions in lecture. He indulges in almost every single question, which slows down lecture tremendously. It's great he wants to resolve any unanswered questions, but it's just too many. (It's also evident that some students ask questions just to make them seem smarter to the professor, but that's another concern.)
I feel like the professor can fix this by setting expectations for questions at the beginning of the course. If you feel like your question helps everyone in class, feel free to ask it in lecture. If not, ask it during office hours.
Overall though, great professor.
I love Tonmoy Monsoor. Super knowledgeable TA, always willing to help during discussion, holds great review sessions.
A great course with a great lecturer and TAs. The lectures are well prepared and Prof. Kao is really good at teaching. He's happy to stop anytime and answer your questions. TAs are very helpful in the discussions and OH. The exams are fair, and do please attend the midterm and final review held by the TA! The topics are very similar to what will appear in the exam so you definitely should spend enough time reviewing these topics. If you are interested in the neuroscience and have a strong knowledge base of probability, linear algebra and Python, the course is a perfect choice. A little bit of matrix calculus is involved but truse me, they just look scary.
40% 6 homeworks, 25% midterm, 35% final.
As all the other reviews state, this class is goated. I petitioned for it to count as a BioE major field elective. For all the non EE people thinking about taking this class: you gotta at least be familiar with the pre-reqs for this class or ur gonna get rekt by all the probability and linear algebra.
Kao is great! Learned a lot. HW's can be tough/time consuming, but mosty because numpy doesn't always behave the way you expect. Dealt with influencers trying to disrupt class very professionally. Generally just a happy guy that seems super down to share what he knows.
I first want to mention that I took this class as a UCLA Extension student. I took it because I was bored to death in UCLA Extension's Data Science program and this class didn't disappoint. I would argue this is the best Machine Learning / Deep Learning class I ever took! The class is hard (be prepared to study a lot) but also incredibly rewarding.
This course has a strong focus on understanding the foundations of Deep Learning so that it isn't a black box anymore. It very often touches the mathematical background of Deep Learning so make sure you are familiar enough with calculus and linear algebra before you hop in. You will be working with tensor sized derivatives a lot and assignments are not coding only!
Assignments are hard but manageable. Overall you will be tasked with either mathematically solving for machine learning problems (e.g find optimal parameters for a noisy linear regression) or manually implementing neural networks in an efficient way. It takes time so make sure not to work last minute.
Besides UCLA Extension I'm a french master's student in Data Science (my course credits this year transfer back to France) and the presence of TAs, discussion sessions and a discussion forum was very new to me. I personally went through the course trying to figure out the homeworks on my own with no help. It makes it harder but you can still manage with enough time.
I also want to highlight that Professor Kao does an amazing job at teaching this class. He explains incredibly well, at a good pace, and also answers questions very quickly.
Overall a great course. If you're looking for a rewarding challenge go for it!
Professor Kao really, really cares about learning and is also a great lecturer, one of the best I've had at UCLA by far. I have a little bit of past experience with ML but Kao's slides and lectures made my understanding so much better, and the way the class is structured forces you to engage with the material. The final project is not bad at all and pretty easy to get 100% on if you can put in some time/thought -- even if you do it solo, IMO. The midterm was definitely very scary to me early on, but it's very similar to past midterms and the TAs do their best to help prepare you (I made some silly mistakes and still got an A on it). Finally, you do not need the prereqs to do well in this class as long as you're willing to put in some extra work early on.
Kao is, hands down, the best professor in the ECE department. His lectures are clear and engaging, and manage to break difficult concepts down into understandable chunks. He provides excellent slides, both annotated from class and unannotated originals, which are wonderful for studying. Kao is absolutely a subject matter expert, since the course focuses on research advances that he was a part of. He can answer literally any question on his lecture material. Seriously, this is what a proper college class should feel like.
A probability prerequisite (not necessarily ECE 131A, but any equivalent class) is absolutely required, and you may struggle without it. Much of the second section of the class focuses on poisson processes, and a course in probability is essential. It would also be helpful to have some knowledge of Python beforehand, since the homeworks generally assume it. However, you don't need any knowledge of electrical engineering at all. There's a tiny section on equivalent circuits in the first part of the course, but you don't need any background knowledge to understand it.
This class is a lot of work. Kao isn't kidding when he tells you that in the first lecture. The homeworks took a long time each, even though there are only 6 of them. They're a mixture of written math solutions and Python coding in Jupyter notebooks. The homeworks are pretty well spaced out, so there's plenty of time to complete them, and the TAs provide exceptional help during discussions (seriously, don't skip discussions. The TAs practically solve homework problems sometimes). Kao gives four "late days" across all the homework, which is an exceptionally generous grading policy.
The tests are difficult, but generally the class average is very good (attribute that to Kao's exceptional teaching abilities). He posts plenty of practice tests beforehand, and the TAs host a long review session for each test, so there is plenty of practice material. Both the midterm and the final had a bonus question for extra credit, but the bonus questions are generally harder than the rest of the test.
Despite the workload of the course, I would absolutely recommend it (and for CS majors, you can petition it to count as a CS elective). This course was one of the best courses I've taken at UCLA, primarily because of Professor Kao. It's a genuine pleasure to take his courses. Even if you have little interest in neuroscience or brain-machine interfaces, you will probably still find this course more engaging than most of the other courses offered at UCLA solely because of Professor Kao.
Lectures are clear and slides are provided.
Deep learning results and certain concepts are interesting but theory is lacking.
Homework is pretty boring. It's all just working with matrix dimensions or finding gradients.
Get a good group for the project.
Midterm is difficult.
Like everyone said, Kao is probably one of the nicest professors I've ever met. While some professors care only about research, it's clear that Kao genuinely cares about teaching. He wants us to learn in an intellectually honest way and grasp the material conceptually. He encourages everyone to come to office hours, he encourages people to ask questions, and he is so super approachable. He has a forgiving late day policy and will give class wide extensions if the class expresses a need. He wants everyone in the class to succeed. The material is challenging but he makes it super accessible and it's super interesting.
The class is 40% homework which sounds nice but I found the homeworks to be harder than the tests because they were long and they had us work on content we literally just learned. You MUST start early. I would say to start immediately, as homeworks are usually assigned Thursdays, do as much as you can over the weekend, and then go to the TA's or his office hours during the week to answer questions. It is very possible that you won't be able to do a lot of the homework alone/when you first look at it, but he and the TAs are VERY helpful and will guide you to the right answer. I like to think I'm not an idiot, and I absolutely could not have done these homeworks without going to office hours.
The rest of the class is 25% midterm and 35% final. After the midterm, he said that if we performed better on the final, he would replace our midterm score with our final score (he's super supportive and on the student's side!). As more Fall 2023 reviews come in, I expect people to say the midterm was easy and the final was hard. I don't know that I will completely agree. The midterm was extremely straightforward, as the TAs held a review session before the midterm that had problems that were almost identical to the real midterm. Thus, many people left after 40 minutes, and the median on the midterm was a 92. I think Kao reacted appropriately and adjusted the difficulty of the final to make it more challenging. I also think a lot of people were expecting the final to be just as easy and it was not. The content on the final is just in general more challenging, but we also weren't spoon fed the entire final like we were the midterm. Thus, many people freaked out after the final and said it was super hard, and I don't think this is super accurate. The final did have a much lower average than the midterm (in the 70s I believe) but I just feel like a 92 median is high in general. There was not a single question on the final that was completely out of left field -- I genuinely believe every question was fair game. Thus, main point is: go to office hours, go to TA review sessions, do EVERY SINGLE practice midterm/final/discussion/homework. And make sure you KNOW the material from a CONCEPTUAL STANDPOINT. To study for the final, I went through every single discussion worksheet, homework, and the past 4 years of finals (he provides them god BLESS) and made sure I understood everything. Also, on your cheat sheet, do NOT just put formulas. The tests test HOW YOU APPLY THE CONCEPTS. He will NOT just be like "State the convolution theorem :D (10pts)." So look at the midterm and final answer keys copy solutions of different types of problems, it will really help you out. Good luck, and really take advantage of what an amazing teacher he is!
I love Jonathan Kao. His lectures are very clear—with amazing annotated notes. Concepts that may seem confusing—Kao has a unique ability to make them seem approachable and common sense-like.
In office hours, he is always willing to take questions, talk about the course, or just life in general. I've had great conversations with him regarding the existence of free will. Not many professors are that engaged with their students.
One minor criticism I have of Kao is how he takes questions in lecture. He indulges in almost every single question, which slows down lecture tremendously. It's great he wants to resolve any unanswered questions, but it's just too many. (It's also evident that some students ask questions just to make them seem smarter to the professor, but that's another concern.)
I feel like the professor can fix this by setting expectations for questions at the beginning of the course. If you feel like your question helps everyone in class, feel free to ask it in lecture. If not, ask it during office hours.
Overall though, great professor.
I love Tonmoy Monsoor. Super knowledgeable TA, always willing to help during discussion, holds great review sessions.
A great course with a great lecturer and TAs. The lectures are well prepared and Prof. Kao is really good at teaching. He's happy to stop anytime and answer your questions. TAs are very helpful in the discussions and OH. The exams are fair, and do please attend the midterm and final review held by the TA! The topics are very similar to what will appear in the exam so you definitely should spend enough time reviewing these topics. If you are interested in the neuroscience and have a strong knowledge base of probability, linear algebra and Python, the course is a perfect choice. A little bit of matrix calculus is involved but truse me, they just look scary.
40% 6 homeworks, 25% midterm, 35% final.
As all the other reviews state, this class is goated. I petitioned for it to count as a BioE major field elective. For all the non EE people thinking about taking this class: you gotta at least be familiar with the pre-reqs for this class or ur gonna get rekt by all the probability and linear algebra.
Kao is great! Learned a lot. HW's can be tough/time consuming, but mosty because numpy doesn't always behave the way you expect. Dealt with influencers trying to disrupt class very professionally. Generally just a happy guy that seems super down to share what he knows.
I first want to mention that I took this class as a UCLA Extension student. I took it because I was bored to death in UCLA Extension's Data Science program and this class didn't disappoint. I would argue this is the best Machine Learning / Deep Learning class I ever took! The class is hard (be prepared to study a lot) but also incredibly rewarding.
This course has a strong focus on understanding the foundations of Deep Learning so that it isn't a black box anymore. It very often touches the mathematical background of Deep Learning so make sure you are familiar enough with calculus and linear algebra before you hop in. You will be working with tensor sized derivatives a lot and assignments are not coding only!
Assignments are hard but manageable. Overall you will be tasked with either mathematically solving for machine learning problems (e.g find optimal parameters for a noisy linear regression) or manually implementing neural networks in an efficient way. It takes time so make sure not to work last minute.
Besides UCLA Extension I'm a french master's student in Data Science (my course credits this year transfer back to France) and the presence of TAs, discussion sessions and a discussion forum was very new to me. I personally went through the course trying to figure out the homeworks on my own with no help. It makes it harder but you can still manage with enough time.
I also want to highlight that Professor Kao does an amazing job at teaching this class. He explains incredibly well, at a good pace, and also answers questions very quickly.
Overall a great course. If you're looking for a rewarding challenge go for it!
Professor Kao really, really cares about learning and is also a great lecturer, one of the best I've had at UCLA by far. I have a little bit of past experience with ML but Kao's slides and lectures made my understanding so much better, and the way the class is structured forces you to engage with the material. The final project is not bad at all and pretty easy to get 100% on if you can put in some time/thought -- even if you do it solo, IMO. The midterm was definitely very scary to me early on, but it's very similar to past midterms and the TAs do their best to help prepare you (I made some silly mistakes and still got an A on it). Finally, you do not need the prereqs to do well in this class as long as you're willing to put in some extra work early on.
Kao is, hands down, the best professor in the ECE department. His lectures are clear and engaging, and manage to break difficult concepts down into understandable chunks. He provides excellent slides, both annotated from class and unannotated originals, which are wonderful for studying. Kao is absolutely a subject matter expert, since the course focuses on research advances that he was a part of. He can answer literally any question on his lecture material. Seriously, this is what a proper college class should feel like.
A probability prerequisite (not necessarily ECE 131A, but any equivalent class) is absolutely required, and you may struggle without it. Much of the second section of the class focuses on poisson processes, and a course in probability is essential. It would also be helpful to have some knowledge of Python beforehand, since the homeworks generally assume it. However, you don't need any knowledge of electrical engineering at all. There's a tiny section on equivalent circuits in the first part of the course, but you don't need any background knowledge to understand it.
This class is a lot of work. Kao isn't kidding when he tells you that in the first lecture. The homeworks took a long time each, even though there are only 6 of them. They're a mixture of written math solutions and Python coding in Jupyter notebooks. The homeworks are pretty well spaced out, so there's plenty of time to complete them, and the TAs provide exceptional help during discussions (seriously, don't skip discussions. The TAs practically solve homework problems sometimes). Kao gives four "late days" across all the homework, which is an exceptionally generous grading policy.
The tests are difficult, but generally the class average is very good (attribute that to Kao's exceptional teaching abilities). He posts plenty of practice tests beforehand, and the TAs host a long review session for each test, so there is plenty of practice material. Both the midterm and the final had a bonus question for extra credit, but the bonus questions are generally harder than the rest of the test.
Despite the workload of the course, I would absolutely recommend it (and for CS majors, you can petition it to count as a CS elective). This course was one of the best courses I've taken at UCLA, primarily because of Professor Kao. It's a genuine pleasure to take his courses. Even if you have little interest in neuroscience or brain-machine interfaces, you will probably still find this course more engaging than most of the other courses offered at UCLA solely because of Professor Kao.
Lectures are clear and slides are provided.
Deep learning results and certain concepts are interesting but theory is lacking.
Homework is pretty boring. It's all just working with matrix dimensions or finding gradients.
Get a good group for the project.
Midterm is difficult.